
Overview of Research Themes
The Valeri Lab develops statistical methods and computational tools for causal inference with the interdependent goals of improving our understanding of social and biological phenomena, of guiding medical decision making and of reducing health disparities, towards better Health for all. We develop statistical and computational approaches that blend causal inference and machine learning principles to fully integrate complex data that we can obtain from different sources and technologies. In particular, we use information available through smartphone devices, electronic medical record data, clinical trials and cohort studies. Below a description of our main areas of research.
01. MECHANISMS explaining complex exposure effects and health disparities
Mediation and Interaction are causal mechanisms that can explain biological and social phenomena and health disparities. We need to take into account the totality of the factors to understand the impact of complex public health interventions. Explaining why and how complex social and environmental exposures affect health outcomes and health disparities using causal machine learning (CML) can help in this direction. This work is funded by an R01 award from the National Institute of Aging (NIA) R01AG077518 and ongoing collaborations with the University of Pennsylvania and Johns Hopkins University.
02. Sensitivity analyses for Missing data AND Measurement error
Causal inferences rely upon complex and at times untestable assumptions. These assumptions include: no unmeasured confounding, no selection bias, no measurement error, positivity, SUTVA, transportability. Statistical tools to fully explore the impact of violation of these assumptions are crucial. This work is funded by an R01 award from the National Institute of Aging (NIA) R01AG077518.
03. Causal inference for smartphone STUDIES in EARLY PSychosis
Statistical learning from personal, human, and social data brings excitement for novel discoveries as well as a number of methodological challenges and privacy concerns in the field of Psychiatry. Ethical alongside Causal and Statistical principles need to guide scientific discovery to support medical decision making in the era of digital Psychiatry. This work is funded by a Career Development Award from the National Institute of Mental Health (NIMH) K01MH118477.
CML to study the health effects of metal mixtures in Bangladesh
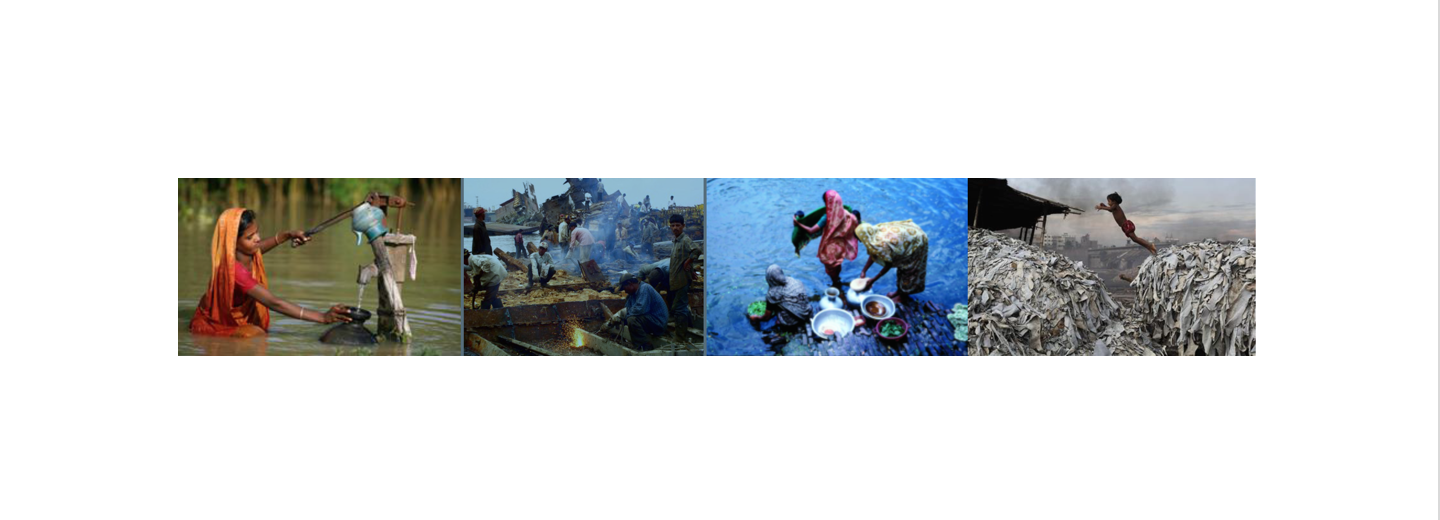
01. Causal Mechanisms
Causal mediation and interaction analyses are methods of causal inference relevant for comparative effectiveness research, evaluating and improving policy recommendations, and explaining biological mechanisms. Formulating causal contrasts and models for the investigation of mechanisms when exposure, outcome and mediator are high dimensional and measured in continuous time results in both theoretical and methodological challenges. We develop approaches to overcome these challenges by combining statistical learning principles of dimension reduction and the causal inference framework. We develop automated software to disseminate the use of statistical approaches and enhance reproducibility (bkmr and CMAverse, for example). With our collaborators at the Departments of Psychiatry, Neurology, Environmental Health Sciences, and Social Sciences primarily based at Columbia University and Harvard University, we apply these approaches primarily towards two endeavors. We investigate mechanisms explaining the effect of environmental mixtures on health. We also quantify the role of health care access and behavioral/environmental factors in explaining racial and socio-economic disparities in health. See the publications section if you are interested in this research!
02. Bias Analysis
Causal inferences rely upon both testable and untestable assumptions. Violation of positivity, no unmeasured confounding and transportability are particularly critical. Measurement error and selection bias, can as well threaten the conclusion of a causal analysis. We develop sensitivity analyses techniques to assess the impact of violations of causal and statistical assumptions deriving non parametric bias results for measurement error, unmeasured confounding and selection bias. We also develop data fusion approaches to use effectively information from external sources to adjust for these biases. See the publications section if you are interested in this research!
03. Causality in Digital Psychiatry
Technological driven change can lead to improvements in medical decision making if we have the ability of learning causal relationships. Mobile devices allow to collect complex data in continuous time either passively or with active participation of the study subjects. The field of Psychiatry is experiencing a revolutionary phase in which statisticians are called to play a critical role to leverage the new richness of this data. Our goal is to develop rigorous statistical approaches for the analysis of observational smartphone based studies to facilitate the discovery of behavioral targets of interventions for the treatment in schizophrenia. At the Lab we develop interactive visualization tools for multi-sensor high-dimensional dense data streams to facilitate quality control checks and hypothesis generation. We develop statistical methods for the estimation of the causal effects of treatments and behaviors in continuous time in N-of-1 psychiatry studies in the presence of pervasive and potentially not at random missing data in both active and passive data streams. This work is funded by the National Institute of Mental Health.
Interactive Dashboard for mHealth data visualization in clinical and research settings
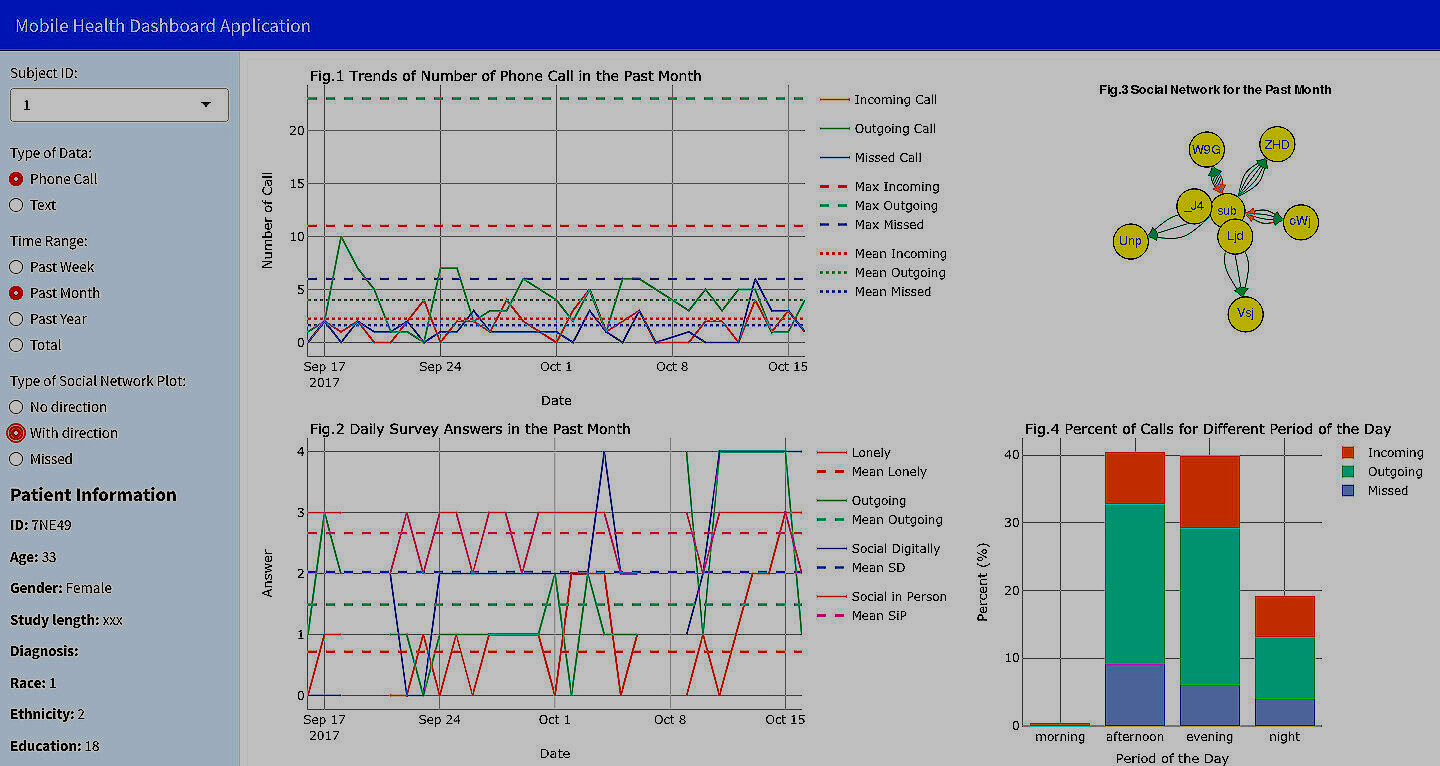
Software
The Valeri Lab is committed to develop open access analytic tools for a broad audience. Below the list of SAS, STATA and R commands available for download and under development. Please, email me if you have any question!
SAS macros mediation and proc causalmed for parametric mediation analysis
STATA command med4way for parametric mediation analysis
R package CMAverse for reproducible mediation analysis (see the tutorial here, we welcome your feedback!)
R package causalbkmr - Bayesian Kernel Machine Regression for causal mediation analysis, time varying exposures and confounding, missing data imputation.
R package rstanmed for sensitivity analyses for time-varying confounding in mediation analysis
R package ssmimpute for missing data imputation of non-stationary time series in mHealth